Share this article
Data mining and artificial intelligence are two technologies that are transforming the way we live and work.
With data mining, we extract useful information from the data generated by the organization, uncovering hidden patterns or trends, while with AI, we create systems that learn and adapt to make autonomous decisions without human intervention.
Next, we will explore the details that encompass the world of data mining and in which business sectors these technologies are already being used. Keep reading and find out everything…
What is data mining?
It is a computer-based process or technique that seeks to discover patterns, trends, and relationships that are often not visible to the naked eye and are hidden within large volumes of data sets.
Its goal is to extract valuable information from the vast amount of data generated by a company, in order to make informed decisions based on the findings.
What are the types or models of data mining?
The types of mining can be used according to the needs of each company, the data, and the purpose of mining. They are classified as predictive, descriptive, and prescriptive.
- Predictive: uses business intelligence to predict trends or future behavior. For example, usage trends, returns, purchases, etc., to make informed decisions based on this data in order to improve organizational efficiency.
- Descriptive: this model detects patterns, similarities, or groupings to understand the characteristics of the analyzed data. It can also identify trends in user behaviors.
- Prescriptive: this model is used to create recommendations or make suggestions.
The difference between the three types of models is quite clear: descriptive describes the past, predictive predicts the future, and prescriptive provides recommendations or actions.
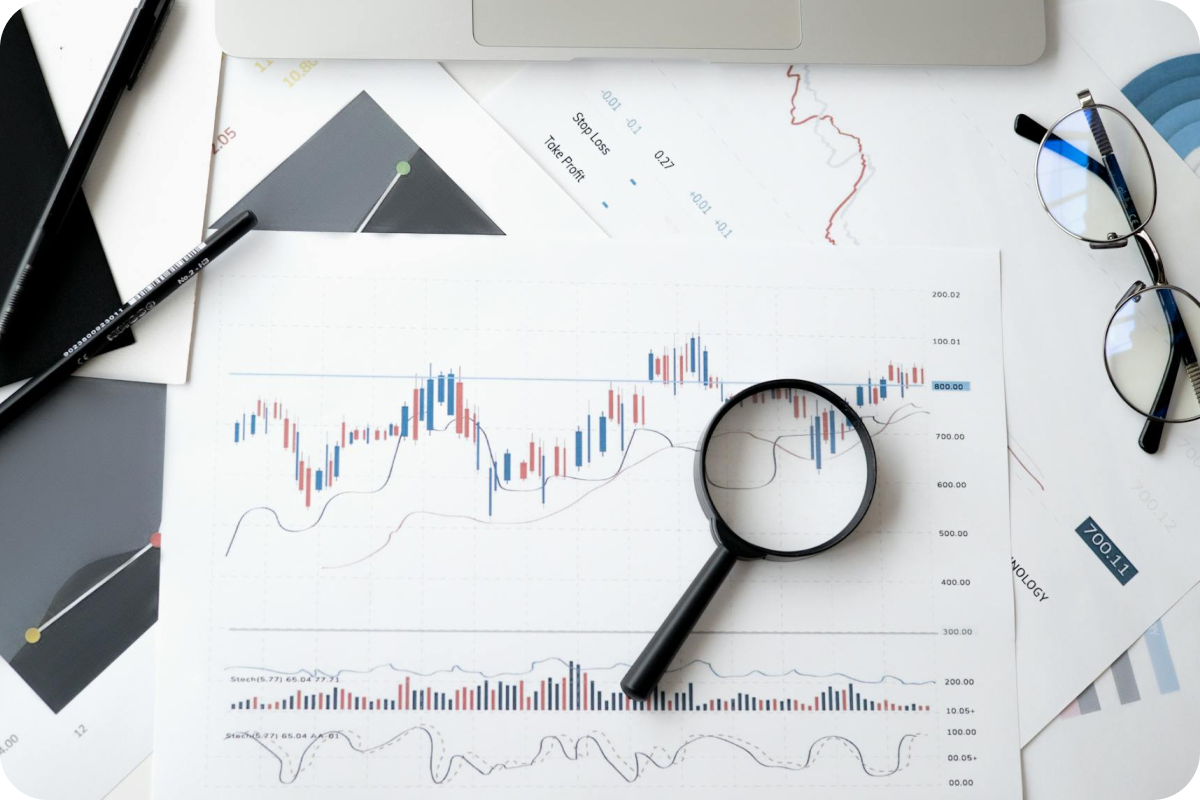
Data mining encompasses the process of discovering patterns, relationships, or hidden information in large and complex datasets.
Data mining techniques
- Data Mining Techniques Clustering: This technique is responsible for grouping data with similar regularities or features, encapsulating results that exhibit the same behavior, such as segmenting customers by life stage or types of purchases made, etc.
- Association: This technique seeks to find relationships between two sets of data that apparently have nothing in common or no apparent relationship, identifying the point of convergence between the two data sets. It is also used to compare new events with existing ones and confirm the variables to which these attributes are connected. This technique is widely used to measure consumer behavior.
- Classification: Algorithms are trained to classify data into different categories. This technique allows for projecting how certain information will be reflected in the future and helps make business or strategic predictions based on it. With this technique, attributes are collected for your company and classified according to their relevance, for example, loyal customers as opposed to new customers arriving at the company.
- Clustering: This grouping brings together various data points based on their similarities. It differs from classification in that it cannot distinguish data into specific categories but can identify patterns in their similarities.
- Sequence and Path Analysis: Here, patterns or a series of events that occur in a sequence are discovered.
What is the data mining process like?
To begin implementing data mining in the company, we must have some clear questions to define the scope of our project.
-
Understanding the Business and Defining the Problem
Knowing what you want to achieve with the project and how far it will go is relevant for making decisions that will guide the process.
All stakeholders in the company should be contacted to identify the problems that will be addressed and how data can solve those problems. Additionally, the project’s limitations, the expected results of the implementation, and how it would impact the company should be determined. Also, what the financial investment will be and potential project difficulties, to create contingency plans if they occur.
Once this information is gathered, decisions are made based on the findings from the collected data.
-
Data Preparation and Understanding
Data preparation involves determining which data should be collected and from which information source it can be extracted to obtain the necessary information.
What hidden correlations exist in this data that can be useful, which data is most accurate for the analysis to be conducted, and whether there are data points that may seem unrelated but are ultimately relevant.
-
Data Exploration and Preparation
During exploration, minimum and maximum values, the mean, and standard deviations are calculated to extract high-quality and reliable data.
At this stage, data that won’t be useful is cleaned or removed, missing data is managed to ensure all necessary details are collected, errors in the data are identified, default values and corrections for such data are determined.
Data integration is also necessary, even for data that may seem unrelated but could provide valuable information. Finally, data should be adapted to the type of technology that will be used for analysis and the specific mining technology that will be applied.
-
Generating the Data Mining Model
According to Microsoft, a data mining model is simply a container that specifies the columns used for input, the attribute being predicted, and parameters that instruct the algorithm on how to process the data.
In other words, at this point, the mining columns need to be established, and the data structure to be used must be created.
Data mining analysts must train machine learning models with small, known data to ensure that the model is well-balanced. They start using the model with unknown data and adjust the software until the desired results are achieved.
-
Evaluating and Verifying Model Effectiveness
Once the data is being extracted, it needs to be checked and its effectiveness verified. Specialists review this data, share it with related areas, gather feedback, and this is where it becomes apparent whether these models provide a solution to the initial problem for which they were created, allowing for adjustments to be made according to the expected outcome of the process.
-
Implementation
In the implementation process, the software is shared with other areas that will also extract data. Specialists train these areas in data extraction and instruct them on how to use the model, providing guidance to these new team members and taking responsibility for maintaining data mining in good working order.
Finally, they must create reports to send to top management, share the results with clients, and improve business processes.
Difference between Data Mining and Machine Learning
Data mining and machine learning are related but not the same. Both are used in the field of data science to analyze and extract useful information from data sets, but they have slightly different approaches and objectives.
- Data mining encompasses the process of discovering patterns, relationships, or hidden information in large and complex data sets. It involves exploring historical data to find valuable insights without necessarily making future predictions.
Statistical and visualization techniques are used to identify trends, patterns, and correlations in the data. Data mining can be used for tasks such as customer segmentation, fraud detection, market analysis, and more. It does not always involve building predictive models.
- Machine learning, on the other hand, focuses on developing algorithms and models that enable computers to learn patterns and perform specific tasks without being explicitly programmed. Its goal is to train predictive models that can make predictions or decisions based on data.
How to Automate Your Tasks with AI and Data Mining?
To automate tasks with artificial intelligence and data mining, patterns and trends are used to build machine learning models and algorithms that allow machines to process data and make decisions without human intervention.
AI can be used to automate tasks, identify complex patterns, and make informed decisions.
Areas where AI and data mining can be used
- Finance: It is used to detect financial fraud, analyze credit risks, and predict market trends.
- E-commerce: This is one of the areas where it is most commonly used because companies can predict product demand, optimize prices according to customers, and recommend products. .
- Manufacturing and Quality: These areas can optimize manufacturing processes, prevent defects, and reduce production costs.
- Telecommunications: They manage networks and detect call fraud.
- Government and Public Administration: Implementing data mining helps make data-driven decisions, improve the management of public resources, and detect fraud in social programs.
- Transportation and Logistics: It enables better supply chain management, route planning, and maintenance scheduling.
Conclusion:
Data mining and AI represent powerful tools for innovation and problem-solving in an increasingly data-driven world, and their influence will continue to grow in the years to come.
References
https://latam.kaspersky.com/resource-center/definitions/data-mining
https://www.astera.com/es/tipo/blog/Las-10-mejores-t%C3%A9cnicas-de-miner%C3%ADa-de-datos/