Share this article
AI-ready data is essential to harness the promise of AI efforts. Understand what it means and follow these five steps to prepare. AI-ready data means your data must be representative of the use case, including every pattern, error, outlier, and unexpected occurrence needed to train or execute an AI model for a specific purpose.
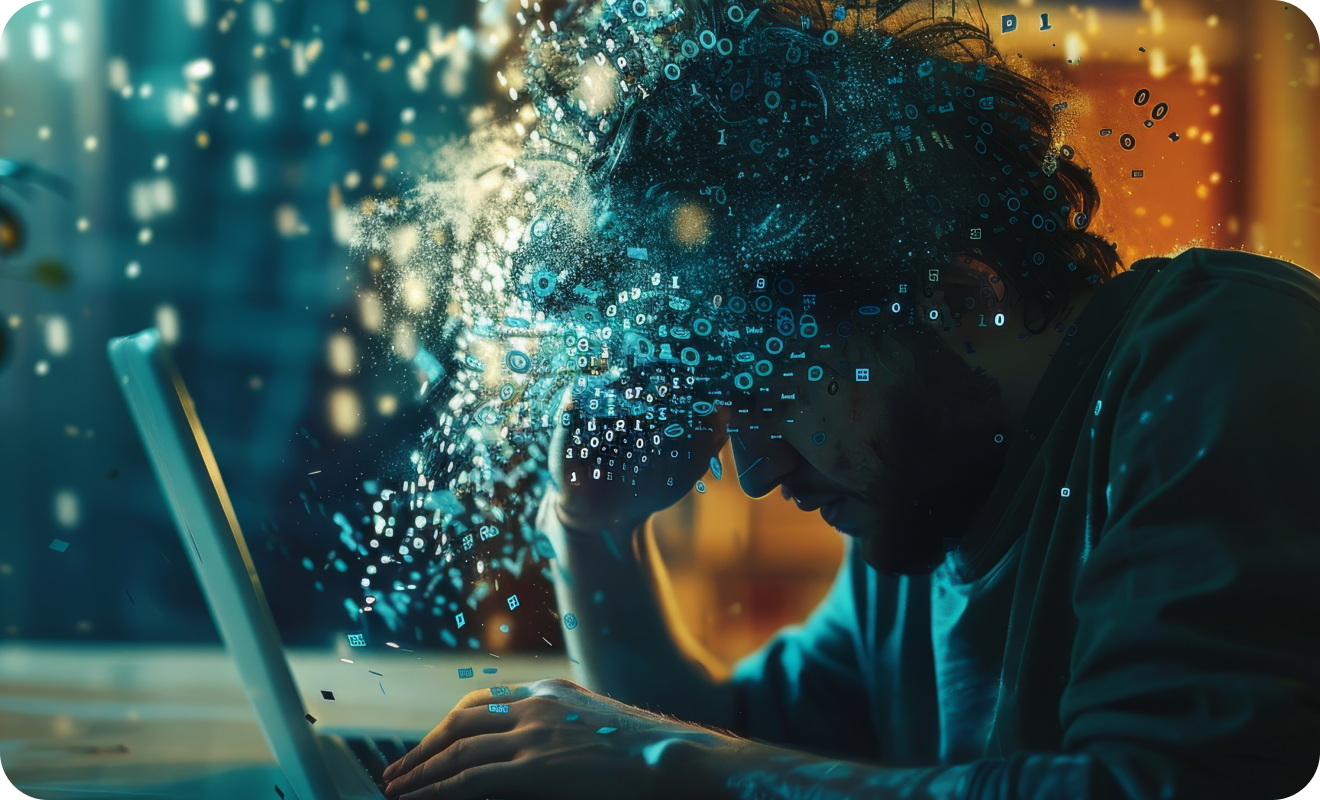
It is a process and practice based on the availability of metadata to align, qualify, and govern the data.
How do we prepare all our data for AI?
There is no way to make data AI-ready in general or in advance. Preparing data for AI depends on how it will be used. For example, very different datasets would be needed to create a predictive maintenance algorithm and to apply GenAI to business data.
If our data is high quality, is it AI-ready?
“High-quality” data – according to traditional data quality standards – does not equate to AI-ready data. When thinking about data in the context of analytics, for example, it is expected to remove outliers or clean the data to support human expectations. However, when training an algorithm, it will require representative data. This can also include low-quality data.
AI-ready data has specific requirements: use a roadmap to stay on track.
Data and analytics leaders must demonstrate that their organization’s data is ready to be used in an increasing number of AI initiatives, but there are significant differences between the requirements for AI-ready data and traditional data management. To bridge that gap, Gartner recommends the following steps:
- Evaluate your data needs based on AI use cases.
- Present the requirements to the board of directors and get approval.
- Evolve data management practices.
- Expand the data management ecosystem.
- Expand and manage.
This roadmap will ensure that your data is ready for use in the specific AI initiatives you plan to carry out and keeps stakeholders aligned on what it truly means for data to be AI-ready.